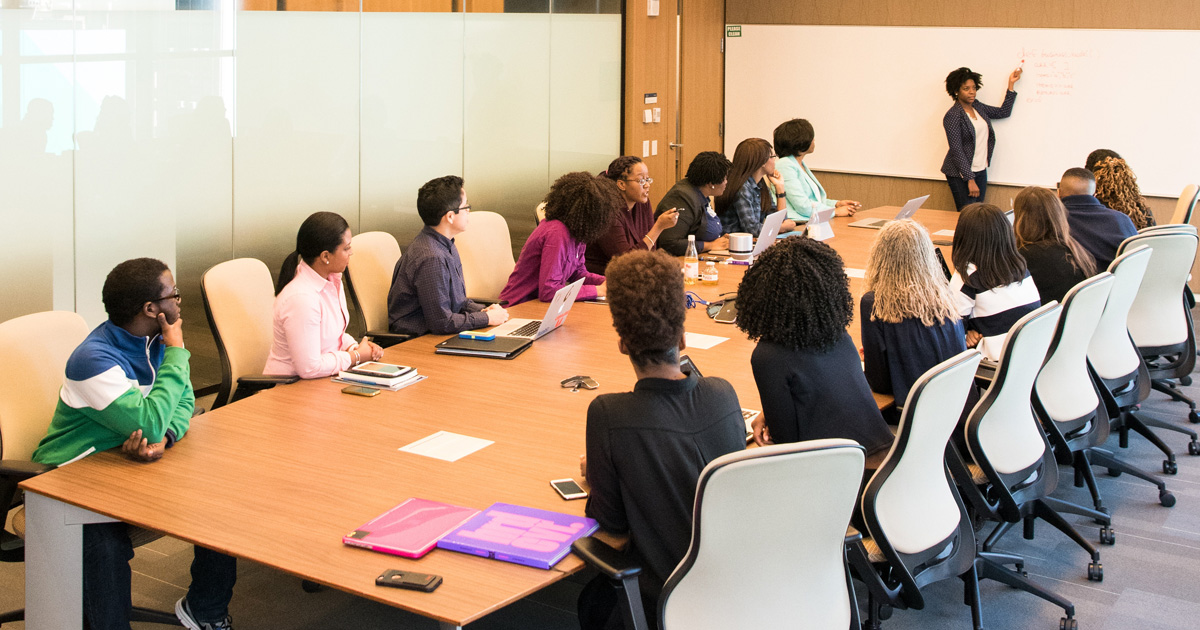
In a previous blog we explored what Machine Learning is and how it is utilized to solve certain types of business problems that humans either cannot solve or don’t because it is too costly or time consuming. From predictively queuing a show for a video subscriber to watch next to mapping the building numbers for every street in France, Machine Learning offers a viable solution. But where do you start?
Identify the Challenge
The power of Machine Learning comes from its process. That process should be driven by an objective that is quantifiable, achievable, and valuable to the business. Ask the question: “What is a capability the business is lacking?” The answers will vary, but will always highlight a lack of capabilities; predicting default or identifying new customers, or improving automation of tasks, triaging issues, or facilitating decision making. A single machine learning solution can have huge value for a business, such as identifying fraud in electronic trading, or detecting comorbid diseases in patients. Once the objective is identified, then the right skill sets are required to apply a Machine Learning approach.
Assemble the Right Team
The most common role that comes to mind when considering Machine Learning is Data Scientist. However, they alone cannot provide all the skills necessary for a successful solution. Machine Learning requires data management and infrastructure, as well as and the ability to evaluate results within the business context. The Data Scientist is key to the analytical modeling portion, but a multidisciplinary team of technical and business domain experts is also needed. A typical Machine Learning team should include the following types of members:
- Domain Expert – Has a deep understanding of the specific business, industry or problem domain. One of the greatest challenges to getting the most out of machine learning is properly understanding the problem, it’s context or the end objective. The domain expert ensures the other team members understand the business value of various approaches and outcomes.
- Platform or Tools Specialist – Understands the platforms, architectural considerations, best practices, and performance tuning for the technology infrastructure and ecosystem. Specifically, key for optimizing and integrating data pipelines in the production environment as well as solution development. Sometimes the best machine learning attempts fail, not due to poor strategy but lack of consideration for computational needs.
- Data Engineer – Performs data acquisition, data modeling, integration, and data pipeline optimization using relevant platform ecosystem tools. Assesses data quality and models the data to support analytical processing. No company on earth has analytics ready data, but machine learning cannot proceed with anything less.
- Data Scientist – Understands analytical model strengths, short comings, and appropriateness for application to different problems. Has advanced training and broad experience with data analysis algorithms, statistical and mathematical techniques. Interprets the findings and communicates the accuracy and strength of the predictive analysis.
- Product or Data Visualization Specialist – Uses visualization tools or creates custom infographics that demonstrate the analytical findings in the context of business questions. Tells a story with the data and addresses why the findings matter and what actions to take. The visualization specialist is often the translator to the business for the machine learning team.
It’s common for the members of a Machine Learning solution team to have skills that cross these five categories, thus allowing for smaller team size, but it’s near impossible to find one person who possesses all of these skills with the necessary depth for an effective solution. Once the complete team is assembled, they are ready for the next step; quickly delivering value through a collaborative process. Stay tuned for our next blog on the collaborative process!